Navigating the Mirage: The Quest for Hallucination-Free AI
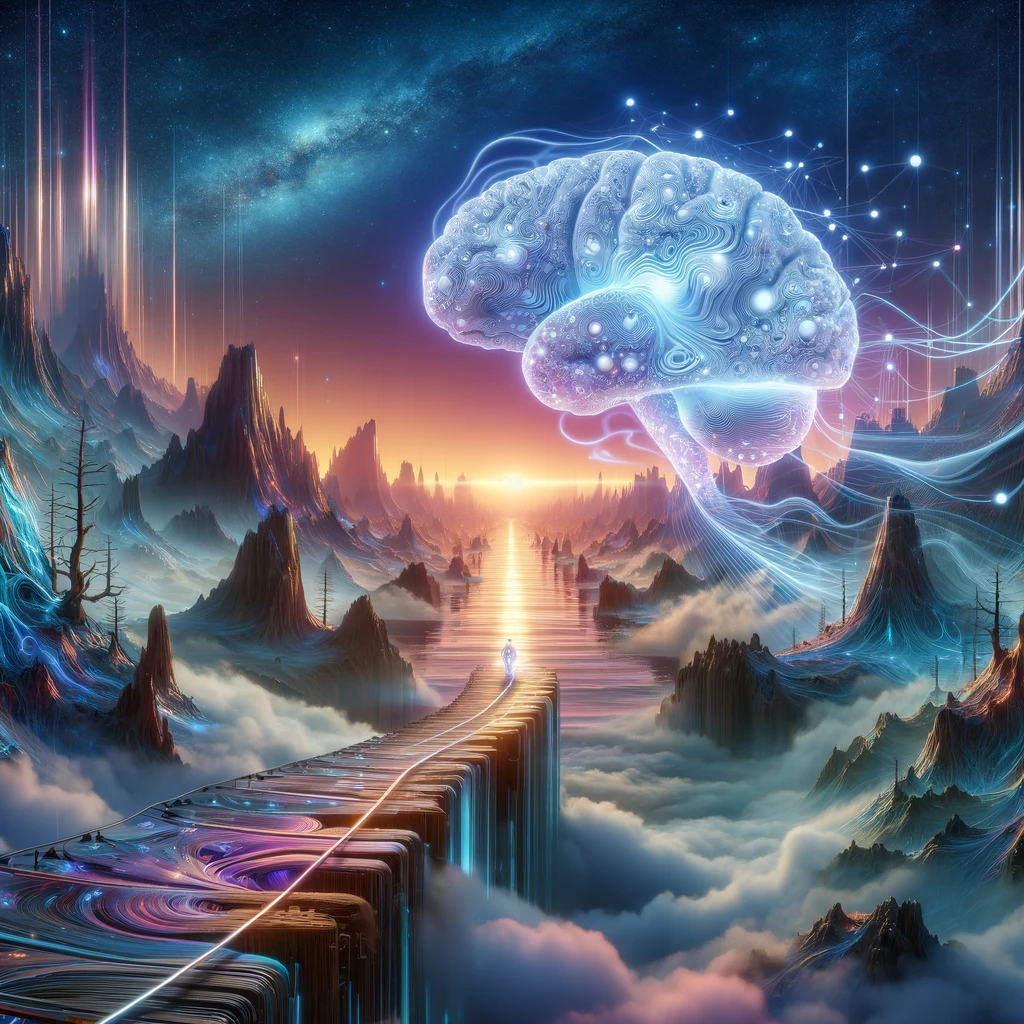
In the rapidly evolving landscape of artificial intelligence (AI), the term “hallucination” has transcended its traditional psychiatric connotations, embedding itself into the lexicon of AI development and ethics. In this context, AI hallucinations refer to instances where AI systems generate false or misleading information, often with a high degree of confidence. This phenomenon raises significant concerns, particularly as AI becomes more integrated into decision-making processes in healthcare, finance, legal systems, and beyond. This article explores the concept of AI hallucinations, the implications of striving for a “hallucination-free” AI, and the ethical, technical, and societal challenges involved.
Understanding AI Hallucinations
AI hallucinations occur when machine learning models, particularly those based on large language models (LLMs) or generative models, produce information that is not grounded in their training data or real-world facts. These inaccuracies can range from minor inaccuracies to significant fabrications, often presented with a misleading aura of credibility. The root causes are multifaceted, including overfitting, biases in training data, and the inherent limitations of current AI architectures in understanding context or verifying the truthfulness of their outputs.
The Quest for Hallucination-Free AI
The pursuit of a “hallucination-free” AI represents an ambitious goal of developing systems that can reliably produce accurate, factual, and contextually appropriate responses. Achieving this would not only enhance the reliability of AI applications but also bolster public trust in AI technologies. However, this endeavor introduces several challenges:
Technical Challenges
Eliminating hallucinations requires advancements in AI models’ ability to discern and adhere to factual accuracy, context awareness, and the application of external knowledge bases for real-time fact-checking. Developing such capabilities involves significant research in natural language processing, knowledge representation, and model interpretability, among other areas.
Ethical and Societal Implications
Striving for hallucination-free AI also raises ethical questions. For instance, who determines what constitutes a “fact,” especially on contentious or evolving issues? Additionally, there’s the risk of overcorrecting, where attempts to prevent hallucinations might lead to overly conservative AI that hesitates to provide useful information or insights in ambiguous situations.
Governance and Standards
Establishing standards and governance frameworks for AI accuracy and truthfulness is crucial. This involves defining benchmarks for acceptable levels of accuracy, transparency in how AI systems make determinations, and mechanisms for accountability when inaccuracies occur.
Strategies for Mitigating AI Hallucinations
Addressing AI hallucinations requires a multi-pronged approach:
- Enhanced Training and Validation: Improving the quality and diversity of training data and employing rigorous validation techniques to test for hallucinations across a wide range of scenarios.
- Fact-Checking Mechanisms: Integrating real-time fact-checking into AI systems, either through external databases or by cross-referencing within the model’s own knowledge base.
- Transparency and User Education: Providing clear explanations of how AI systems generate their outputs, including the limitations and potential for inaccuracies, can help users critically assess AI-generated content.
- Ethical AI Development Practices: Adopting ethical guidelines that prioritize accuracy, transparency, and accountability in AI development can guide efforts to minimize hallucinations.
- Community Engagement and Oversight: Involving a broad community of stakeholders, including ethicists, domain experts, and end-users, in oversight and continuous improvement processes can ensure diverse perspectives inform efforts to reduce hallucinations.
Conclusion
The quest for hallucination-free AI embodies the broader challenges of responsible AI development: balancing technical innovation with ethical considerations and societal impact. While achieving completely hallucination-free AI may remain an aspirational goal, ongoing efforts to mitigate these issues are crucial for the trustworthy advancement of AI technologies. As we navigate this complex terrain, the focus must remain on developing AI that enhances human decision-making with integrity, accuracy, and respect for the nuances of human knowledge and understanding.